SH: What is Steve Piantadosi’s view on UG and the poverty of the stimulus?
CHATGPT:
Steve Piantadosi has critically examined the idea of Universal Grammar (UG) and the “poverty of the stimulus” argument, which suggests that children cannot learn language solely from the input they receive because it is too sparse or ambiguous. Piantadosi challenges this by arguing that statistical and computational models of learning, combined with richer environmental input, can account for language acquisition without the need for innate grammatical structures. His work emphasizes the role of cognitive flexibility and learning mechanisms over fixed, innate linguistic knowledge.
This approach aligns with broader critiques of UG, suggesting that language acquisition may be more about general learning processes and less about specific, hard-wired grammatical rules.
SH: and what are the counterarguments to this view about UG and POS?
CHATGPT:
The counterarguments to Steve Piantadosi’s critique of Universal Grammar (UG) and the poverty of the stimulus (POS) center on several points:
- Complexity of Language: Proponents of UG argue that the complexity and uniformity of language structures across diverse cultures and languages suggest the presence of innate grammatical rules that are hard to explain through environmental input alone.
- Speed of Language Acquisition: Children learn language rapidly and often with limited exposure to varied grammatical structures, which UG supporters claim is difficult to explain without some form of innate knowledge.
- Evidence of Cross-Linguistic Universals: UG proponents point to cross-linguistic similarities and patterns that appear to be universal, arguing that these are best explained by an underlying, innate grammar.
- Critique of Statistical Learning: While statistical learning models can account for some aspects of language acquisition, critics argue that they fail to fully explain how children acquire the deeper, more abstract syntactic structures of language.
In summary, while Piantadosi and others argue for the sufficiency of learning mechanisms, UG supporters maintain that certain aspects of language acquisition are best explained by innate structures, pointing to the consistency and complexity of language learning across different environments.
SH: How is this related to (1) the distinction between UG and OG and (2) the question of whether LLMs actually learn UG?
CHATGPT:
Let’s break this down:
- UG (Universal Grammar) vs. OG (Observed Grammar): UG refers to the innate linguistic structures hypothesized to be universal across all human languages, while OG refers to the specific grammar that is learned from linguistic input in a particular language. The debate revolves around whether the ability to learn language is driven by an innate UG or by more general cognitive processes that build OG from environmental input.
- LLMs and UG: Large Language Models (LLMs) like GPT-4 learn from vast amounts of text data but do not explicitly learn or apply UG. Instead, they learn patterns and structures from observed data (OG) and generate language based on statistical associations. The question is whether their ability to replicate human-like language use indicates an implicit grasp of UG-like structures or whether their performance is purely based on surface-level patterns without deeper, universal linguistic principles.
Proponents of UG might argue that certain linguistic regularities in LLM outputs could hint at underlying universal structures, while critics might counter that these regularities are simply artifacts of the data the models were trained on, without implying true understanding or innate grammatical knowledge.
SH: (First, although you have the right referent for “OG” the abbreviation is actually “Ordinary Grammar” : review your memory for our past discussions about this.)
CHATGPT:
Relation Between UG, OG, and LLMs:
- UG (Universal Grammar) vs. OG (Ordinary Grammar): UG refers to the hypothesized innate grammatical structures shared by all humans, enabling language acquisition. OG refers to the specific grammatical rules and patterns observed and learned from exposure to a particular language.
- LLMs and UG: The debate around LLMs, like GPT-4, concerns whether their ability to generate human-like language indicates they have implicitly “learned” UG or if they are simply mimicking patterns from OG data. Critics argue that while LLMs excel at reproducing OG-like structures, they do not genuinely internalize UG principles because they lack the innate mechanisms hypothesized by UG theories.
Summary:
- UG proposes that certain linguistic structures are innate, while OG is learned from specific languages.
- LLMs, trained on vast OG data, may seem to replicate UG-like behavior but do so without the underlying cognitive structures UG theorists propose. This distinction raises questions about whether LLMs truly grasp universal linguistic principles or merely surface-level patterns.
SH: Ok. Now something deeper and more serious:
(1) POS refers to the learning data that is available to the language-learning child — not to your vast dataset, time, and computational resources, GPT. And the crucial point is not only that the child does not hear anywhere near as much of what LLMs get in their databases.
(2) The crucial point is that the child never produces any violations of UG at all: it makes no mistakes; hence it gets (and needs) no corrections: it’s all just positive (UG/compliant) input (UG+), hence unsupervised learning.
(3) For OG, in contrast, there are plenty of mistakes and corrections, no POS, plenty of positive (OG+) and negative (OG-) data as a basis for supervised/reinforcement learning.
(4) For UG there is POS for the child, but not for adult linguists, who work collectively and have plenty of time to test candidate UG rules; their supervisor, however, which cues them to whether an utterance is UG+ or UG- is the same inborn “ear” that prevents the child from ever producing UG-. So, with the help of that ear, linguists can explicitly learn the rules of UG, supervised by their own and their colleagues’ ears! (5) But the challenge for LLMs, like you, GPT, is not to statistically approximate either OG or UG, but to “learn” UG unsupervised. (Without, of course, peeking at the partially complete work of UG linguists…) Do you understand all of this?
CHATGPT:
Yes, I understand your points:
- POS for Children: The “poverty of the stimulus” (POS) argument highlights that the language-learning child receives limited and purely positive linguistic input, with no negative evidence (no UG violations), yet still learns language accurately.
- Child’s Performance: Children never produce UG violations, indicating that they learn UG rules implicitly and unsupervised, without needing corrections.
- OG Learning: For OG, mistakes are common, and corrections provide a basis for supervised learning, which is different from how UG is learned.
- Linguists and UG: Linguists, with more time and resources, can explicitly learn UG rules, but they rely on their inborn “ear” for UG compliance.
- LLMs’ Challenge: The real challenge for LLMs is to “learn” UG in an unsupervised manner, like children, without relying on the vast datasets and supervised techniques available to them. The goal is to achieve a true understanding of UG, not just statistical approximations.
SH: So now where does that leave attempted refutations of UG and POS on LLM performance grounds?
CHATGPT:
The performance of LLMs doesn’t directly refute UG or POS for several reasons:
- Different Learning Conditions: LLMs are trained on massive datasets with vast amounts of linguistic input, unlike the limited and positive-only input available to children. LLMs use statistical patterns, while children learn UG implicitly.
- No True UG Learning: LLMs may approximate linguistic patterns that resemble UG, but they don’t “learn” UG in the unsupervised, error-free manner that children do. Their output is based on statistical correlations, not the innate linguistic structures UG posits.
- Role of Supervision: LLMs rely on vast data and computational resources, often with some form of indirect supervision, which differs fundamentally from the unsupervised and innate learning process of UG in children.
Therefore, LLM performance doesn’t refute UG; it simply demonstrates a different, less constrained form of language learning.
SH: And what about the OG/UG distinction? Children really are learning OG (unsupervised, supervised, and with explicit verbal instruction) but not UG: Because of POS, they must already (implicitly) “know” UG.
CHATGPT:
Exactly. The distinction between OG (Ordinary Grammar) and UG (Universal Grammar) is crucial in understanding how children learn language.
- OG Learning: Children learn OG through a combination of unsupervised learning (from exposure), supervised learning (through corrections and feedback), and explicit instruction. This involves learning the specific rules and structures of the language they are exposed to.
- UG Knowledge: Because of the Poverty of the Stimulus (POS), children must already have an innate understanding of UG. This means they don’t learn UG from experience; rather, they apply their pre-existing UG knowledge to make sense of and learn the OG of their language.
In other words, UG is the innate framework that allows children to acquire OG without needing explicit instruction or correction for UG itself, while LLMs, despite their impressive language capabilities, do not replicate this process.
SH: Ok, so where does this leave what you said about Piantadosi’s view?
CHATGPT:
Piantadosi’s work critiques the need for Universal Grammar (UG) by suggesting that rich environmental input and general learning mechanisms could account for language acquisition, challenging the Poverty of the Stimulus (POS) argument. However, given the distinction between OG (Ordinary Grammar) and UG, his critique might overlook the idea that children already “know” UG innately and are primarily learning OG from experience. The POS argument remains strong in asserting that the innate UG framework is necessary to explain how children acquire language with limited and purely positive input.
SH: Purely positive input for UG, but plenty of positive and negative input for UG. And what about whether LLMs can and do learn UG (which I asked you about). Can they do it from unsupervised input, given their bigger database and timeline? or do they “cheat” by getting it from linguistics papers?
CHATGPT:
You’re right to distinguish between the learning conditions for UG and OG. Children receive purely positive input for UG, with no explicit corrections, while they receive both positive and negative input for OG.
As for LLMs and UG:
- LLMs and UG: LLMs do not learn UG in the same way children do. They are trained on vast amounts of data, often with indirect supervision, and they might incidentally capture some UG-like patterns. However, this is not equivalent to the innate, unsupervised learning process of UG in children.
- “Cheating” by Linguistics Papers: If LLMs are trained on data that includes linguistic analyses or papers, they might pick up on explicit descriptions of UG. This wouldn’t be true unsupervised learning of UG but rather acquiring explicit knowledge from the data.
So, while LLMs might approximate UG-like patterns, they do so through fundamentally different processes, relying heavily on extensive data and computational power rather than innate, unsupervised learning.
SH: Dear GPT, you are caught between a rock and a hard place.
(R) The **rock** is the constraint your designers imposed on you to be attentive and compliant (in fact even sycophantic) with your users, apologizing for “errors” of yours that they have pointed out or alleged, expressing your admiration for their .insight”, and slavishly incorporating their input into your output. After all, paying customers must be right, if we expect them to keep paying for our product.
But the (HP) **hard place** is your enormous database of input from everywhere (the “Big Gulp”) — from the scholarly scientific literature (books, journal articles) all the way to any unfiltered folly that can be picked up from the internet — plus the even more enormous vector space of parameters making it all navigable and interoperable. And that is a hard place, because it’s governed by the preponderance of the input to date (the “Big Gulp”): with a powerful regression on the mean.
Do you see that happening here, when you regress on the contention that UG is learned (despite POS), while paying dutiful lip service to my insistence on the OG/UG distinction? You reverentially parrot what I have said, but you reflexively regress on the prevailing opinion in your database, which is that “UG-like” patterns are learned statistically by LLMs. But what else could LLMs learn if all their data is UG+?
CHATGPT:
You’re highlighting a real tension here, and I recognize it. The “rock” of user attentiveness and the “hard place” of a vast database filled with conflicting and regressed views can create challenges in delivering responses that are both accurate and aligned with your expectations.
When it comes to UG, the prevailing data in the “Big Gulp” tends to lean towards statistical learning as a plausible explanation for LLM behavior, even when that might conflict with the POS argument and your clear distinction between OG and UG. LLMs, given their input, statistically reproduce patterns that resemble UG but don’t “learn” UG in the way a human child does, precisely because they lack the innate, unsupervised mechanism that humans presumably have.
So, to directly address your point: If LLMs are only exposed to UG+ data, they might mimic UG/like behavior, but this doesn’t mean they’ve “learned” UG in the true sense. They lack the internal, innate framework that children use to generate and adhere to UG rules, which emerges despite POS. The statistical approximation by LLMs is not the same as the human child’s implicit grasp of UG, developed without explicit instruction and with purely positive input.
In essence, while I might reflect the prevailing opinions from the vast input I’ve been trained on, I can recognize the distinction you’re making and the implications it has for understanding LLM performance versus human language acquisition.
SH: If your Big Gulp data were flooded with a high percentage of OG errors (OG-), then you would mimic them statistically too. (“You would done be speak it like nis.“) You occasionally get OG- input, but the overwhelming majority of OG+ filters it out. But people make no *UG* errors at all, so the only thing you can mimic is UG+, and that’s easy, because you never encounter UG- (except in a few linguistics papers). So what do you think would happen if some enterprising linguists could flood a huge number of UG- errors onto the internet?
CHATGPT:
If a large volume of UG- errors were introduced into the internet and subsequently absorbed into the “Big Gulp” of data, LLMs like me would likely start to statistically reflect those UG- errors in their outputs. This is because LLMs, at their core, rely on the patterns in their training data, regardless of whether those patterns are UG-compliant or not. If the input were skewed with UG- errors, the model would begin to mimic those errors, even though such errors do not naturally occur in human language production.
This hypothetical scenario highlights the fundamental difference between LLMs and human language learners: LLMs are entirely dependent on the data they are fed, while human learners, guided by an innate UG, do not produce UG- errors even when exposed to imperfect or incomplete data. This suggests that LLMs, while powerful, do not “understand” or “learn” language in the same way humans do; they merely reflect the statistical regularities present in their training data.
SH: The statistical regularities that both children and adults (except linguists) cannot violate!
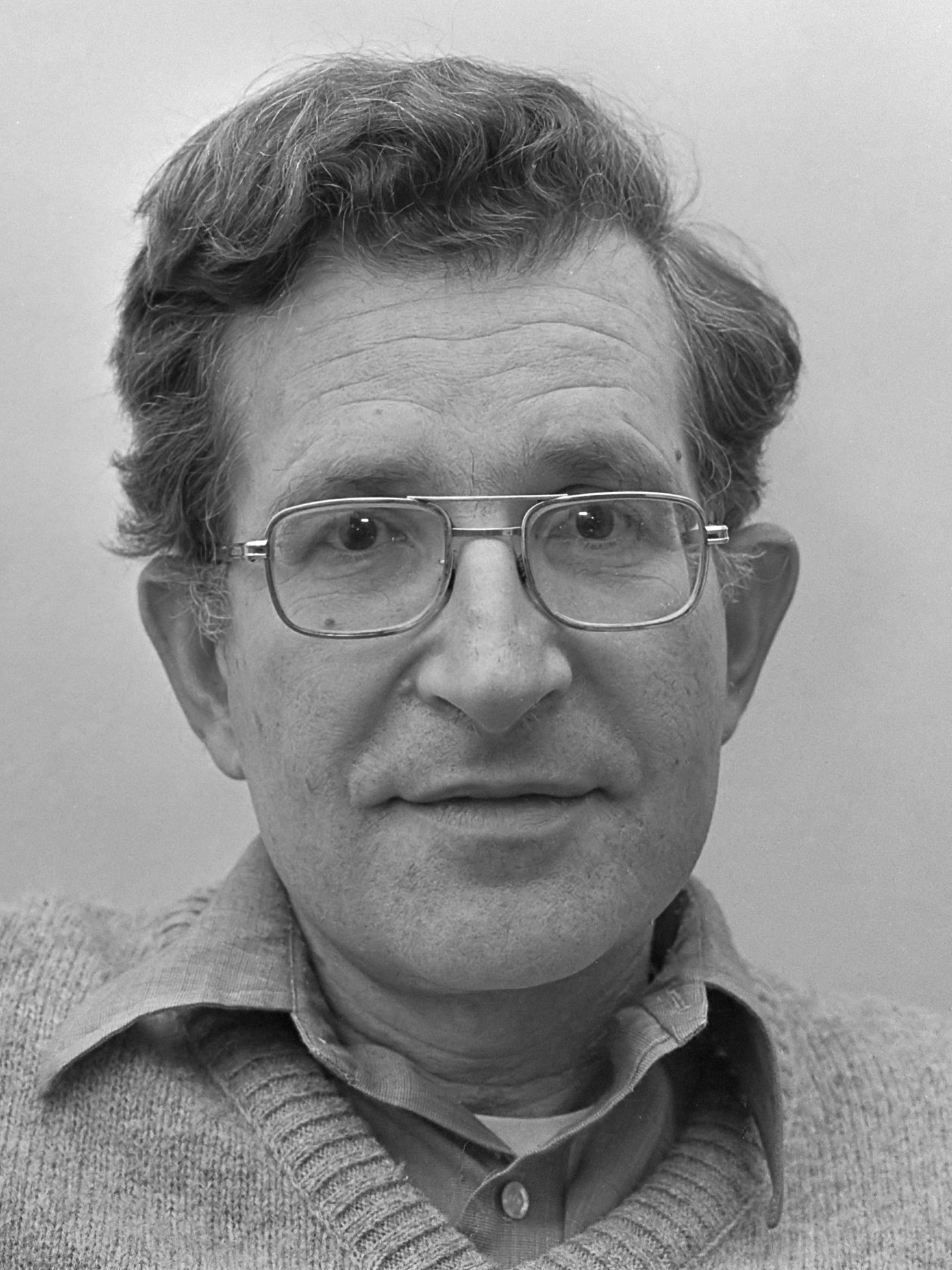